Data analytics is reshaping the healthcare industry, offering powerful insights that improve patient outcomes, streamline operations, and drive cost efficiencies. As healthcare organizations face an ever-growing pool of data, the ability to turn this information into actionable insights is not just an advantage, but a necessity.
This blog explores how data analytics is being applied in healthcare, delving into use cases, technologies, and best practices that ensure organizations can leverage data for innovation and improvement.
The healthcare data landscape
Data in healthcare comes from a wide variety of sources, each offering unique insights that contribute to a holistic view of patient care. These sources include:
- Electronic health records (EHRs): Contain patient history, diagnoses, medications, and more.
- Medical imaging data: MRI, X-rays, and CT scans that contribute to diagnostic accuracy.
- Wearable devices: Offer real-time data on patient health metrics such as heart rate, glucose levels, and physical activity.
- Genomic data: Provides information that can lead to personalized treatment plans.
- Patient-generated health data (PGHD): Inputs from mobile apps and personal health trackers.
Given the vast and complex nature of healthcare data, healthcare data analytics becomes an essential tool to harness its full potential.
Types of analytics in healthcare
Healthcare data analytics can be broadly categorized into three types, each providing different levels of insights and decision-making capabilities:
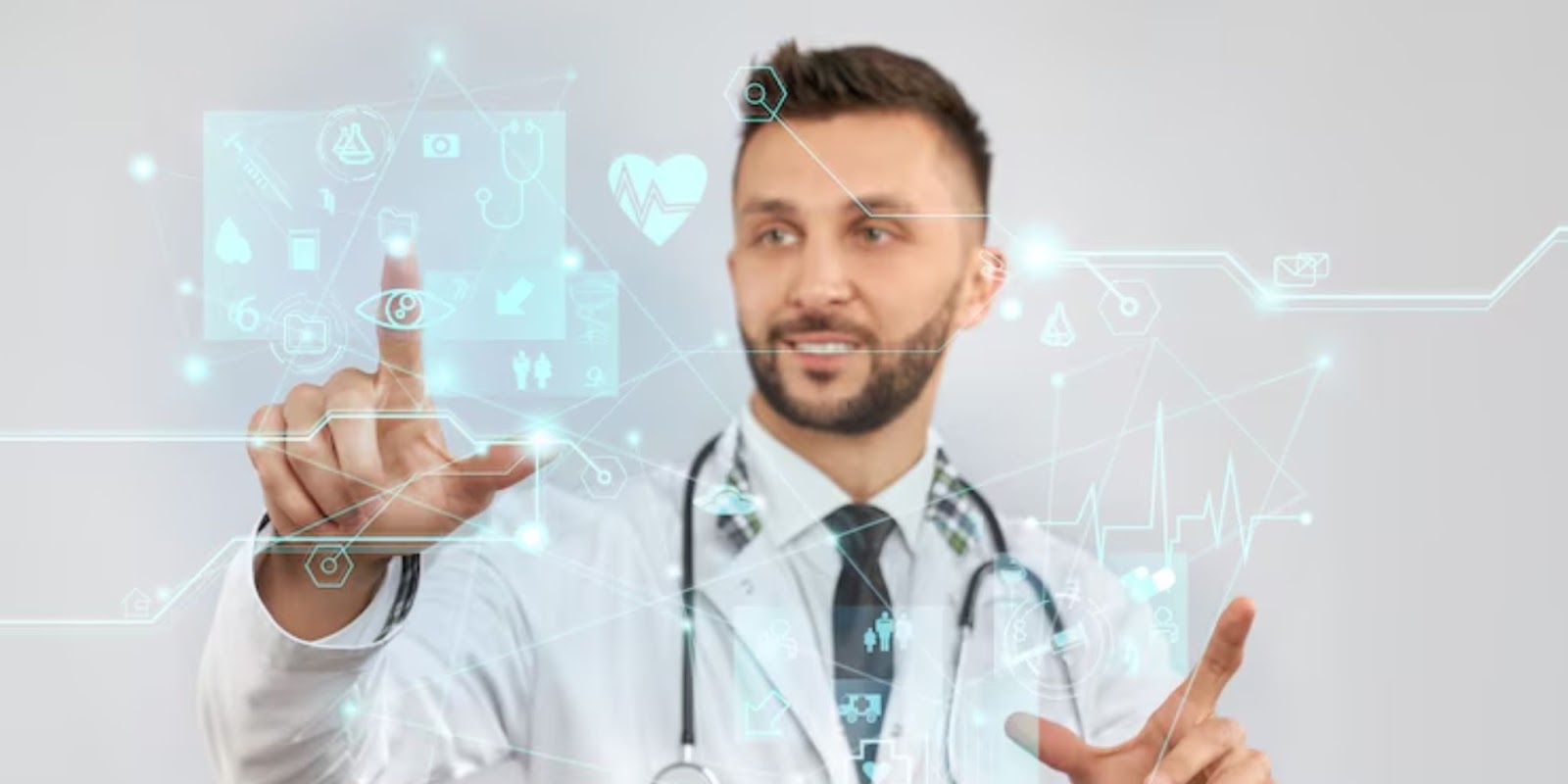
- Descriptive analytics: This involves analyzing historical data to understand trends and patterns, which can inform decisions around resource allocation and patient care improvements. Descriptive analytics forms the foundation of effective decision-making in healthcare systems, as outlined here.
- Predictive analytics: Predictive analytics uses statistical models and machine learning algorithms to forecast future outcomes. In healthcare, predictive models are increasingly being used for preventive care, predicting disease outbreaks, and reducing hospital readmissions.
- Prescriptive analytics: Taking it a step further, prescriptive analytics suggests the best actions to take, often using AI and machine learning to recommend treatment plans or operational changes that can lead to better patient outcomes.
Use cases of data analytics in healthcare
Data analytics has numerous applications in healthcare, transforming the way organizations deliver care, manage resources, and improve patient satisfaction. Below are some of the most impactful use cases:
- Personalized medicine
Through genomic data analytics, healthcare providers can now offer treatments tailored to an individual's genetic makeup. This not only improves treatment efficacy but also reduces the risks of adverse drug reactions. A well-known example is the application of data analytics in oncology, where algorithms help determine the most effective treatments for cancer patients based on their genetic profiles. - Population health management
Analyzing healthcare data on a large scale enables organizations to identify trends and risk factors across specific populations. This allows for proactive interventions, reducing the burden on hospitals and improving patient outcomes. - Operational efficiency
Healthcare facilities use data analytics to optimize scheduling, resource allocation, and supply chain management, which leads to significant cost savings. By applying cloud modernization strategies, organizations can ensure that their data infrastructures are agile and scalable. - Remote patient monitoring
Wearable devices and mobile health apps generate real-time data that can be analyzed to provide continuous patient monitoring. This is especially beneficial for chronic disease management, as it allows healthcare providers to intervene early. The role of AI-powered analytics in remote monitoring is explored in this case study. - Reducing fraud and abuse
Predictive analytics is being employed to detect fraudulent claims by identifying unusual patterns in billing data. This helps in reducing fraud-related losses, a significant issue in the healthcare sector.
The impact of cloud technologies on healthcare analytics
Cloud-based solutions have significantly enhanced the ability to store, process, and analyze vast amounts of healthcare data. With the integration of cloud computing, healthcare providers can leverage massive computational power for data analytics without the limitations of on-premises infrastructure. For small and medium-sized healthcare businesses, cloud solutions enable secure, scalable, and cost-efficient data management.
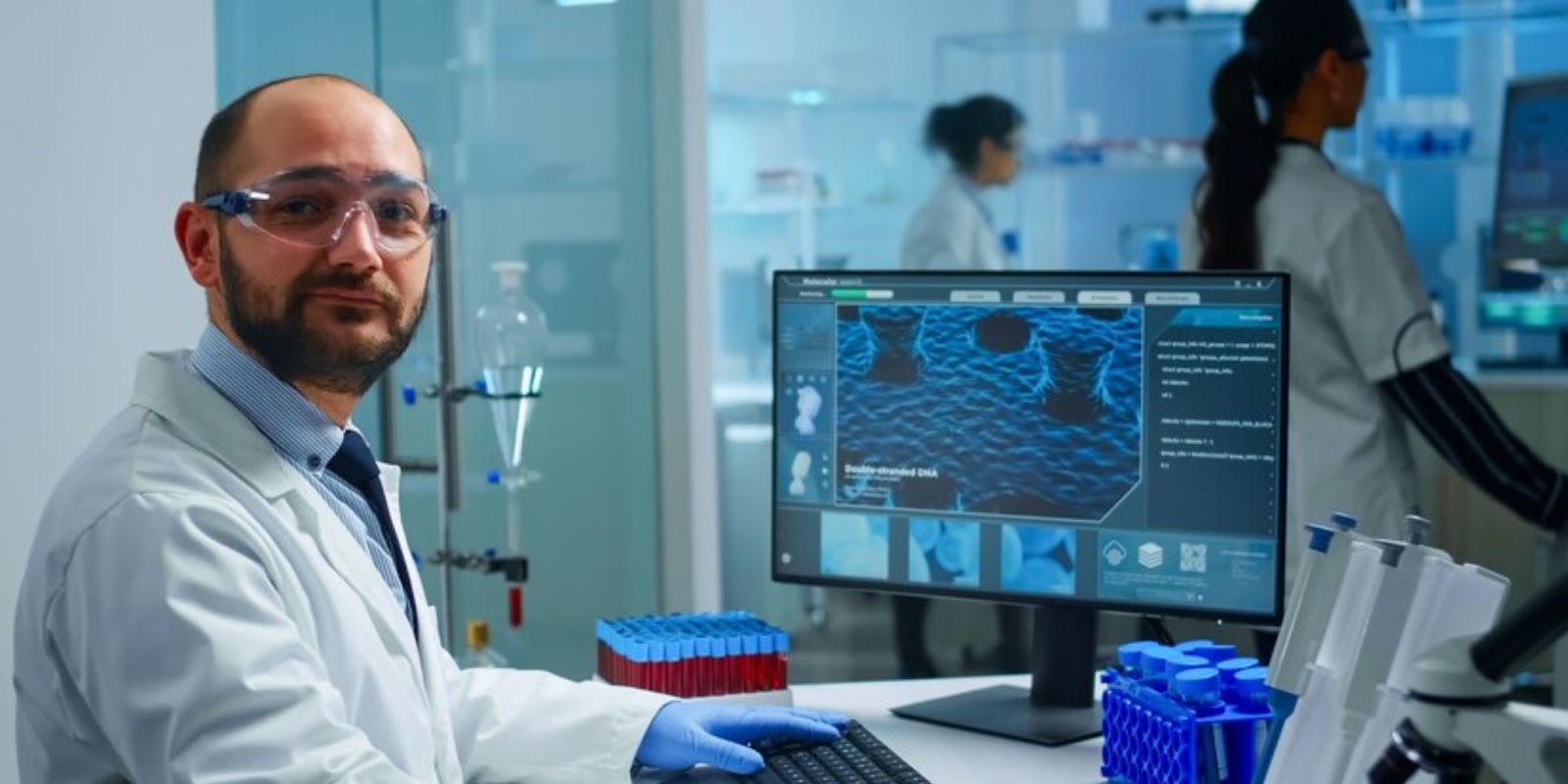
Moreover, cloud modernization opens the door for advanced capabilities like AI and machine learning, facilitating better decision-making and predictive insights. These technologies can be critical in reducing latency in healthcare analytics, thus allowing for faster, more informed decisions in patient care.
Best practices for implementing data analytics in healthcare
While the benefits of data analytics in the healthcare industry are immense, realizing its full potential requires careful planning and execution. Below are some best practices for healthcare organizations:
- Data integration and interoperability
Integrating data from different sources (EHRs, wearables, genomic data, etc.) is crucial for obtaining a comprehensive view of patient health. Ensuring interoperability across systems is key to breaking down silos and enhancing the accuracy of analytics. - Data governance and compliance
The healthcare industry is highly regulated, with strict compliance requirements like HIPAA in the US. Effective data governance policies that ensure data privacy and security are essential for maintaining patient trust and complying with regulatory standards. - Invest in advanced analytics tools
Leveraging advanced technologies like AI and machine learning can unlock new levels of insight and predictive capabilities. Generative AI is an emerging area with potential applications in everything from drug discovery to diagnostic support. - Training and upskilling
Healthcare organizations need to invest in upskilling their workforce to make the most of their data analytics capabilities. Training programs that focus on data literacy and the use of advanced analytics tools can lead to more effective data-driven decision-making.
The bottom line
The growing role of data analytics in healthcare cannot be overstated. From improving patient outcomes and operational efficiency to driving innovation in treatment approaches, data is the lifeblood of the modern healthcare ecosystem. For organizations looking to harness the power of healthcare analytics, understanding the available tools, best practices, and the importance of cloud-based solutions is crucial.
As we move forward, embracing advanced analytics, AI, and cloud technologies will ensure healthcare providers can deliver better care, reduce costs, and foster innovation. If you’re ready to explore how cloud and AI solutions can transform your healthcare data strategy, let us get in touch with our cloud experts.