How AI is writing rules of data analytics
The explosion of data in today’s digital-first economy has transformed data analytics into a vital function for businesses aiming to stay competitive. However, as datasets grow more complex and vast, traditional analytics methods often fall short. Enter Artificial Intelligence (AI)—the catalyst rewriting the rules of data analytics and enabling organizations to extract actionable insights with unprecedented accuracy and speed.
This blog explores how AI is revolutionizing data analytics, uncovering its potential to drive decision-making, innovation, and growth. We’ll also discuss emerging trends, challenges, and actionable strategies to stay ahead in an AI-driven analytics landscape.
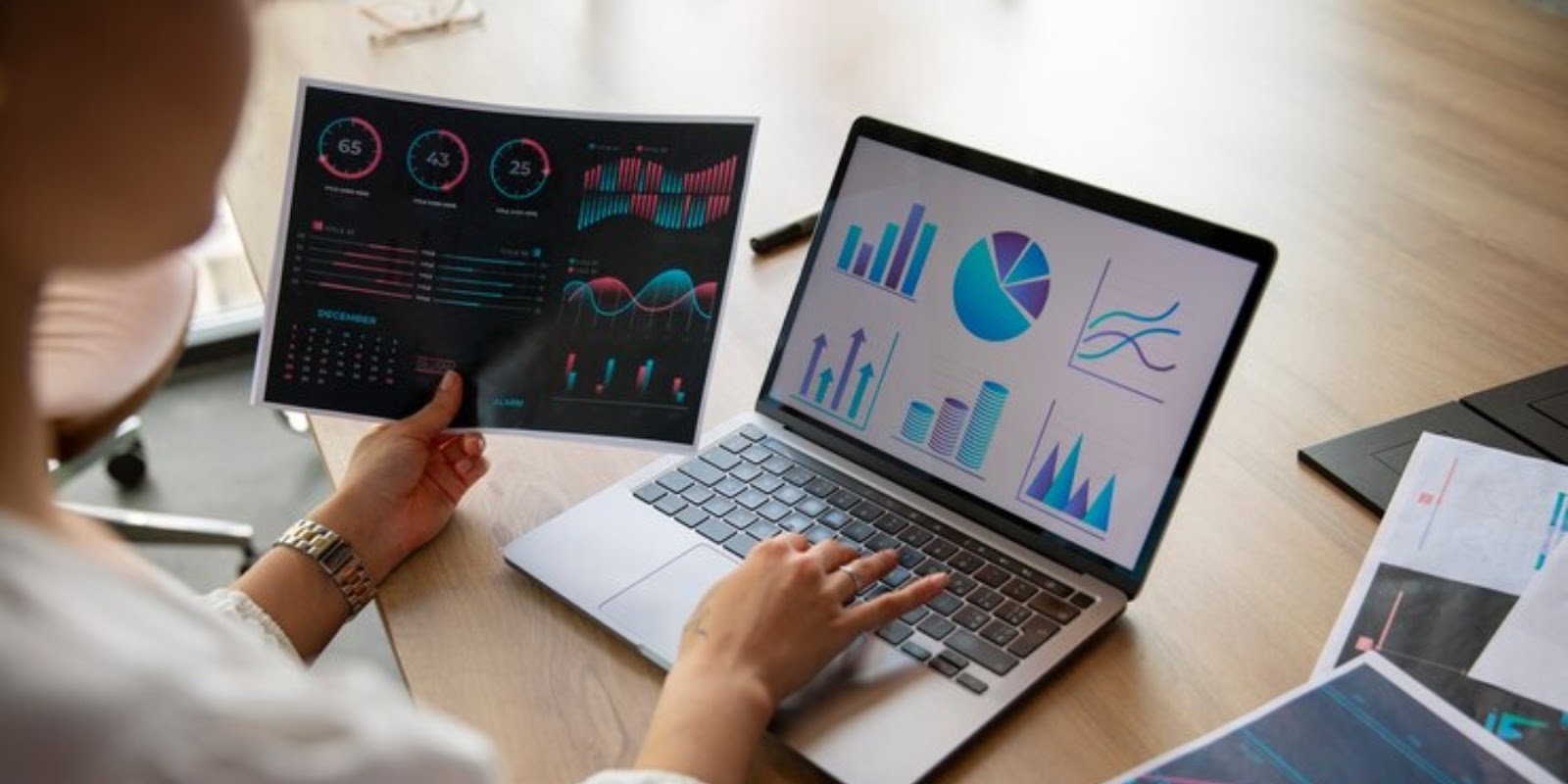
The intersection of AI and data analytics
AI and data analytics are converging to create a transformative shift in how businesses harness data. Unlike traditional analytics, where humans analyze historical data to draw insights, AI introduces automation, predictive capabilities, and self-learning mechanisms to uncover deeper patterns and trends.
From enhancing customer experiences to optimizing operations, AI-powered analytics is shaping the future of decision-making.
AI’s role in modern data analytics
Automating data processing
AI-powered tools automate the tedious aspects of data analytics, such as data cleansing, categorization, and integration.
- AI algorithms can analyze and process data up to 100 times faster than traditional methods (Forbes).
- A global retail giant reduced data preparation time by 80% using AI-based tools, enabling faster insights into customer behavior.
Advancing Predictive Analytics
AI excels at predictive analytics by identifying patterns and correlations within data, allowing businesses to forecast trends and outcomes accurately.
- Companies adopting AI for predictive analytics report a 20-25% increase in revenue generation (McKinsey).
- Financial institutions are using AI to predict market fluctuations and customer creditworthiness, reducing risks and enhancing profitability.
Enhancing decision-making with real-time insights
AI enables real-time analytics, empowering businesses to make informed decisions swiftly.
- 70% of organizations leveraging real-time AI analytics report faster decision-making and increased agility (Gartner).
- An e-commerce platform implemented AI-driven analytics to optimize pricing strategies in real-time, increasing sales by 15%.
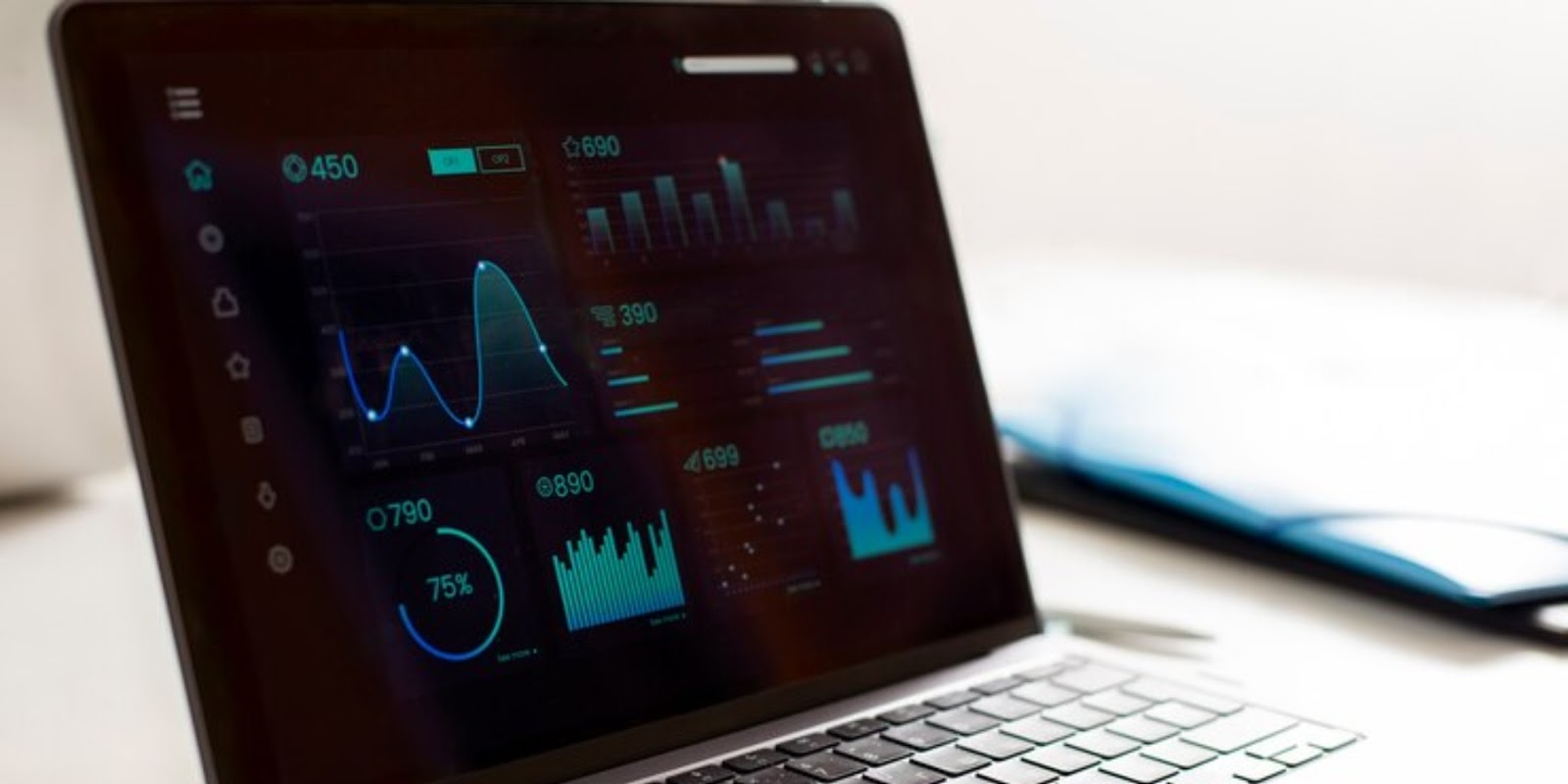
Transformative applications of AI in data analytics
AI-driven business intelligence
AI integrates with business intelligence (BI) platforms to generate intuitive dashboards and actionable insights.
- By combining AI with BI tools, organizations gain a 360-degree view of operations and customer behaviors.
- Integrate AI-powered analytics solutions like Power BI or Tableau to enhance reporting capabilities.
Natural Language Processing (NLP) in analytics
NLP enables AI systems to process unstructured data, such as text and speech, for richer insights.
- Over 80% of enterprise data is unstructured, and NLP tools help organizations tap into this untapped potential (IDC).
- A healthcare provider used NLP to analyze patient feedback, identifying trends that improved service quality and patient satisfaction.
Explore how generative AI consulting can help your organization leverage NLP capabilities.
Generative AI in data visualization
Generative AI transforms raw data into visually engaging and easy-to-understand formats, enabling stakeholders to grasp insights quickly.
- Example: Marketing teams use generative AI to create dynamic dashboards that display campaign performance in real-time.
Emerging trends shaping AI-driven data analytics
Augmented analytics
AI enhances traditional analytics by suggesting actionable insights, guiding decision-making, and automating complex tasks.
- Augmented analytics adoption is expected to grow by 30% annually as businesses seek smarter decision-making tools (Gartner).
AI democratization
AI tools are becoming more accessible, allowing non-technical teams to leverage advanced analytics without needing data science expertise.
- Use Case: Low-code platforms enable marketing and sales teams to run analytics independently, driving faster results.
Explainable AI (XAI)
As AI becomes central to analytics, businesses demand greater transparency into how AI models generate insights.
- Implement XAI frameworks to ensure ethical, transparent, and unbiased AI systems.
Overcoming challenges in AI-driven data analytics
While AI brings immense value, organizations must address certain challenges to unlock its full potential:
- Data Quality: Poor data quality undermines AI analytics. Investing in robust data governance ensures accurate and reliable insights.
- Integration Complexity: Merging AI systems with existing analytics platforms requires careful planning and seamless integration strategies.
- Talent Gap: Organizations often struggle to find AI experts who can manage and optimize analytics processes.
Best practices for implementing AI-driven data analytics
- Invest in Quality Data: High-quality data forms the backbone of effective AI analytics.
- Adopt Scalable Platforms: Use cloud-based analytics solutions to handle growing datasets efficiently.
- Foster Cross-Functional Collaboration: Ensure seamless collaboration between technical and business teams.
- Prioritize Ethical AI: Implement safeguards to address biases and ensure transparency.
A new era for data analytics
AI is rewriting the rules of data analytics, enabling organizations to transform raw data into actionable insights with greater speed and accuracy. By embracing AI-driven solutions, businesses can unlock new opportunities for growth, innovation, and resilience in an increasingly competitive market.
Whether it’s optimizing operations, enhancing customer experiences, or driving innovation, the integration of AI into analytics is no longer optional—it’s essential. Start your journey toward smarter analytics with our data analytics services today.