The integration of Artificial Intelligence (AI) in data analysis is revolutionizing business intelligence, providing unprecedented insights, predictions, and automation capabilities.
According to McKinsey, companies utilizing customer analytics extensively are 23 times more likely to outperform their competitors. This blog explores the transformative impact of AI on data analytics, highlighting key concepts, applications, and future trends.
AI in data analytics
Data analysis has evolved from traditional statistical methods to sophisticated AI-driven techniques capable of handling vast and complex datasets. This section will introduce the importance of AI in data analytics, setting the stage for a deeper exploration of its applications and benefits.
The Evolution of Data Analysis with AI
Traditional Data Analysis vs. AI-Driven Analysis
- Traditional Methods: Traditional data analysis involves manual processes and predefined algorithms, which can be time-consuming and prone to human error. These methods often struggle with large, complex datasets.
- AI-Driven Methods: AI-driven data analysis utilizes machine learning (ML) and deep learning to automatically identify patterns and trends in data. This approach increases accuracy, speed, and scalability, enabling businesses to derive deeper insights from their data.
Example: A retail company might use traditional methods to analyze sales data at the end of each month, while an AI-driven approach could provide real-time insights into sales trends, allowing for quicker decision-making.
Enhanced Predictive Analytics
Predictive analytics uses historical data to forecast future trends. AI enhances this process by:
- Improving Accuracy: AI algorithms can analyze vast amounts of data, identifying subtle patterns and correlations that traditional methods might miss.
- Speeding Up Analysis: AI can process data in real-time, providing timely insights critical for decision-making.
- Automating Predictions: AI models continuously learn from new data, improving their predictive capabilities over time.
Example: A financial institution using AI for predictive analytics can more accurately forecast market trends and customer behaviors, enabling better investment strategies and risk management.
Types of AI in Data Analytics
Beyond generative AI, several other AI types play crucial roles in data analytics. These include:
Machine Learning (ML)
Machine learning is a subset of AI focused on developing algorithms that allow computers to learn from and make decisions based on data. Key aspects include:
- Supervised Learning: Models are trained on labeled data, making predictions based on input-output pairs.
- Unsupervised Learning: Models identify patterns and relationships in unlabeled data, often used for clustering and association tasks.
- Reinforcement Learning: Models learn optimal actions through trial and error, receiving rewards for successful outcomes.
Machine learning techniques empower businesses to make data-driven decisions by uncovering patterns and insights that are not immediately apparent, leading to more informed and strategic actions.
Deep Learning
Deep learning, a subset of ML, involves neural networks with many layers (deep neural networks). It excels in processing unstructured data such as images, audio, and text.
- Convolutional Neural Networks (CNNs): Primarily used for image and video recognition tasks.
- Recurrent Neural Networks (RNNs): Effective for sequential data, such as time-series analysis and natural language processing.
Deep learning's ability to process and analyze complex unstructured data makes it invaluable for tasks requiring high levels of accuracy and detail, transforming industries like healthcare, automotive, and more.
Natural Language Processing (NLP)
NLP is a branch of AI that focuses on the interaction between computers and humans through natural language. Applications include:
- Sentiment Analysis: Understanding and classifying emotions in text data.
- Text Summarization: Automatically generating concise summaries of long documents.
- Chatbots: Providing customer service through automated conversational agents.
NLP allows businesses to automate and enhance their interactions with customers, providing more personalized and efficient service while reducing operational costs.
Generative AI for Data Analytics
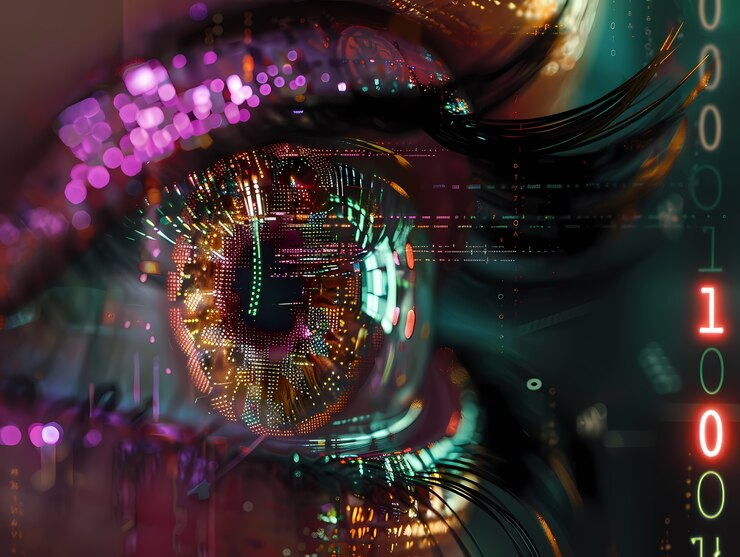
Understanding Generative AI
Generative AI involves algorithms that create new data samples from existing data. This technology is particularly useful for:
- Data Augmentation: Enhancing training datasets by generating synthetic data, improving the robustness of machine learning models.
- Anomaly Detection: Identifying unusual patterns in data that may indicate fraud or system failures.
- Natural Language Processing (NLP): Generating human-like text for applications like chatbots and virtual assistants.
Example: In healthcare, generative AI can create synthetic medical records to train diagnostic models without compromising patient privacy.
Applications of Generative AI
- Healthcare: Generative AI can generate synthetic patient data for training diagnostic algorithms, enhancing their accuracy without violating privacy regulations.
- Finance: It is used to create synthetic financial data, improving fraud detection systems and risk management models.
- Retail: Generative AI helps personalize customer experiences by generating product recommendations based on customer behavior data.
Industry Applications of AI in Data Analytics
Healthcare
AI-driven data analytics in healthcare leads to early diagnosis, personalized treatment plans, and efficient patient management.
- Predictive Diagnostics: AI models analyze medical records and imaging data to predict disease onset.
- Personalized Treatment: AI tailors treatments based on individual patient data, leading to better outcomes.
- Operational Efficiency: AI streamlines administrative tasks and optimizes resource allocation in hospitals.
Finance
AI enhances data analytics in finance by improving fraud detection, risk management, and personalized banking services.
- Fraud Detection: AI systems analyze transaction patterns to identify fraudulent activities.
- Credit Risk Assessment: AI evaluates creditworthiness using diverse data sources, improving loan approval accuracy.
- Customer Personalization: AI offers personalized financial products and services based on customer data.
Retail
Retailers leverage AI for demand forecasting, inventory management, and enhancing customer experiences.
- Demand Forecasting: AI predicts product demand to optimize inventory levels.
- Customer Insights: AI analyzes purchase history and preferences to offer personalized recommendations.
- Supply Chain Optimization: AI uses predictive analytics to streamline logistics and reduce costs.
Manufacturing
AI transforms manufacturing with predictive maintenance and quality control.
- Predictive Maintenance: AI uses sensor data to predict equipment failures and schedule timely maintenance.
- Quality Control: AI analyzes production data to detect defects and ensure product quality.
- Process Optimization: AI improves manufacturing processes by analyzing data from various production stages.
The Future of Data Analytics with AI
The future of AI in data analytics promises even greater advancements. Anticipated developments include:
- Autonomous Data Analysis: AI systems capable of independently analyzing data and making decisions without human intervention.
- Real-Time Analytics: Enhanced real-time data processing capabilities for immediate insights.
- Enhanced Decision Support: More sophisticated AI models providing deeper insights and strategic recommendations.
The Bottom Line
The integration of AI in data analytics is transforming how businesses operate and compete. AI-driven data analysis, enhanced predictive analytics, and innovative approaches like generative AI provide businesses with powerful tools to derive deeper insights, automate complex tasks, and make more informed decisions.
Industries such as healthcare, finance, retail, and manufacturing are witnessing significant benefits, including improved accuracy, efficiency, and personalization. As AI continues to evolve, it will further revolutionize data analytics, offering real-time insights and autonomous decision-making capabilities. Businesses that embrace AI-driven data analytics will gain a competitive edge, leveraging data to drive strategic advantages.
Ready to elevate your data analytics with AI? It’s time for our experts to drive your business forward. Get a free cloud consultation from our experts to transform your data into actionable insights and strategic advantages.