In 2024, data is the cornerstone of decision-making and innovation, making the architecture for storing and managing data crucial. According to a report by Gartner, the global market for data management solutions is expected to reach $122 billion by 2024.
As businesses seek to harness the power of data, understanding the differences between data lakes and data warehouses becomes imperative. This comprehensive guide explores the distinctions, use cases, and benefits of each, helping you make informed decisions about your data architecture.
Jump to the section that discusses the key differences between Data Lake and Data Warehouse in case you know the basics!
What is a Data Lake?
A data lake is a centralized repository that allows you to store all your structured and unstructured data at any scale. You can store data as-is, without having to first structure the data, and run different types of analytics—from dashboards and visualizations to big data processing, real-time analytics, and machine learning.
Key Features
- Scalability: Can handle large volumes of data.
- Flexibility: Supports all data types (structured, semi-structured, and unstructured).
- Schema-on-read: Allows data to be stored in raw form and structured when read.
Data lakes provide a highly scalable and flexible environment for large-scale data storage and analysis, making them ideal for big data applications.
What is a Data Warehouse?
A data warehouse is a centralized repository designed to store and manage large volumes of structured data from multiple sources. It is optimized for querying and reporting, enabling business intelligence activities.
Key Features
- Structured Data Storage: Stores data in a structured format.
- Schema-on-write: Data is processed and structured before storage.
- Optimized for Querying: Designed for fast query performance and data analysis.
Data warehouses are tailored for structured data storage and complex querying, making them suitable for business intelligence and reporting.
Data Lake vs Data Warehouse-Key Differences
While both data lakes and data warehouses serve as data repositories, they cater to different needs and have distinct characteristics. Understanding these differences is crucial for choosing the right solution for your organization.
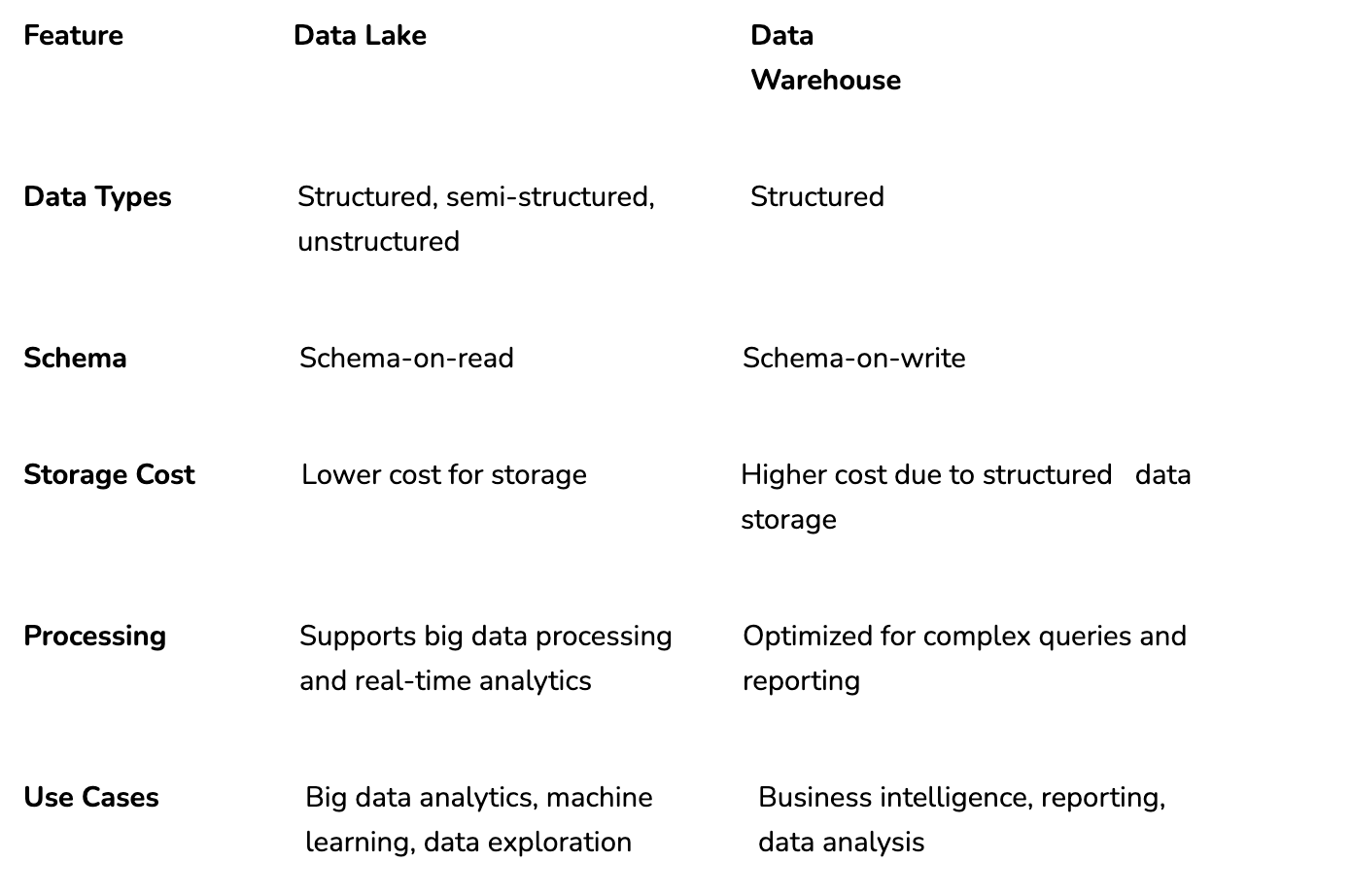
Data lakes and data warehouses are designed for different purposes, and the choice between them depends on your specific data management and analysis needs.
Use Cases for Data Lakes
Data lakes excel in scenarios where large volumes of diverse data need to be ingested, stored, and analyzed. They are particularly useful for big data and machine learning applications.
Common Use Cases
- Big Data Analytics: Handle massive amounts of data from various sources. Implement advanced data analytics solutions on AWS with us!
- Machine Learning: Store raw data for training machine learning models.
- IoT Data Management: Collect and process data from IoT devices.
Data lakes are ideal for environments that require scalability and flexibility to handle vast amounts of diverse data types.
Use Cases for Data Warehouses
Data warehouses are optimized for structured data storage and querying, making them suitable for business intelligence and reporting tasks.
Common Use Cases
- Business Intelligence: Generate insights and reports for decision-making.
- Historical Data Analysis: Analyze historical data trends.
- Data Consolidation: Integrate data from multiple sources for comprehensive analysis.
Data warehouses are best suited for structured data analysis and reporting, providing fast and efficient query performance.
Cloud Data Warehouse Solutions
With the rise of cloud computing, cloud data warehouses have become a popular choice for businesses looking to leverage the scalability and flexibility of the cloud.
Popular Cloud Data Warehouse Solutions
- Amazon Redshift: A fully managed data warehouse service that can handle petabyte-scale data.
Read about modernizing data warehousing with Amazon Redshift here. - Snowflake: A cloud-native data warehouse offering data sharing, scalability, and advanced analytics.
Cloud data warehouses provide a scalable and cost-effective solution for businesses to manage and analyze large volumes of structured data.
Choosing Between Data Lake and Data Warehouse
Deciding whether to use a data lake or a data warehouse depends on your organization's data strategy, use cases, and specific needs.
Considerations
- Data Variety: If you need to store and process various data types, a data lake might be the better choice.
- Query Performance: For fast and complex queries on structured data, a data warehouse is more suitable.
- Cost: Evaluate the cost implications of both storage and processing.
Both data lakes and data warehouses have their strengths and are often used together in a modern data architecture to meet diverse data needs.
The Bottom Line
Understanding the key differences between data lakes and data warehouses is crucial for designing an effective data strategy. By leveraging the strengths of each, businesses can enhance their data management capabilities, drive innovation, and gain valuable insights.
As data continues to grow in volume and complexity, having the right infrastructure in place will be vital for staying competitive in 2024 and beyond.
Explore how you can integrate data lakes and data warehouses into your data strategy. Contact us for a free consultation to learn more about optimizing your data architecture with the latest AWS solutions.