Generative AI has become a cornerstone of modern technological advancements, powering everything from AI-generated art to complex business automation solutions. But how does generative AI actually work? What makes it capable of creating new content, solving complex problems, or even anticipating user needs?
In this comprehensive guide, we will demystify the workings of generative AI by breaking down its components, applications, and the underlying technologies that make it possible. Whether you're a business leader exploring generative AI for innovation or simply curious about the technology's capabilities, this blog will explain how generative AI functions at its core and how businesses can leverage it.
What is generative AI?
Generative AI is a subset of artificial intelligence focused on creating new data, ideas, or content based on existing data. Unlike traditional AI, which classifies or predicts outcomes, generative AI produces entirely new outputs, including text, images, audio, video, and even code.
These outputs are generated by AI models that learn from patterns in the input data to "imagine" new possibilities.
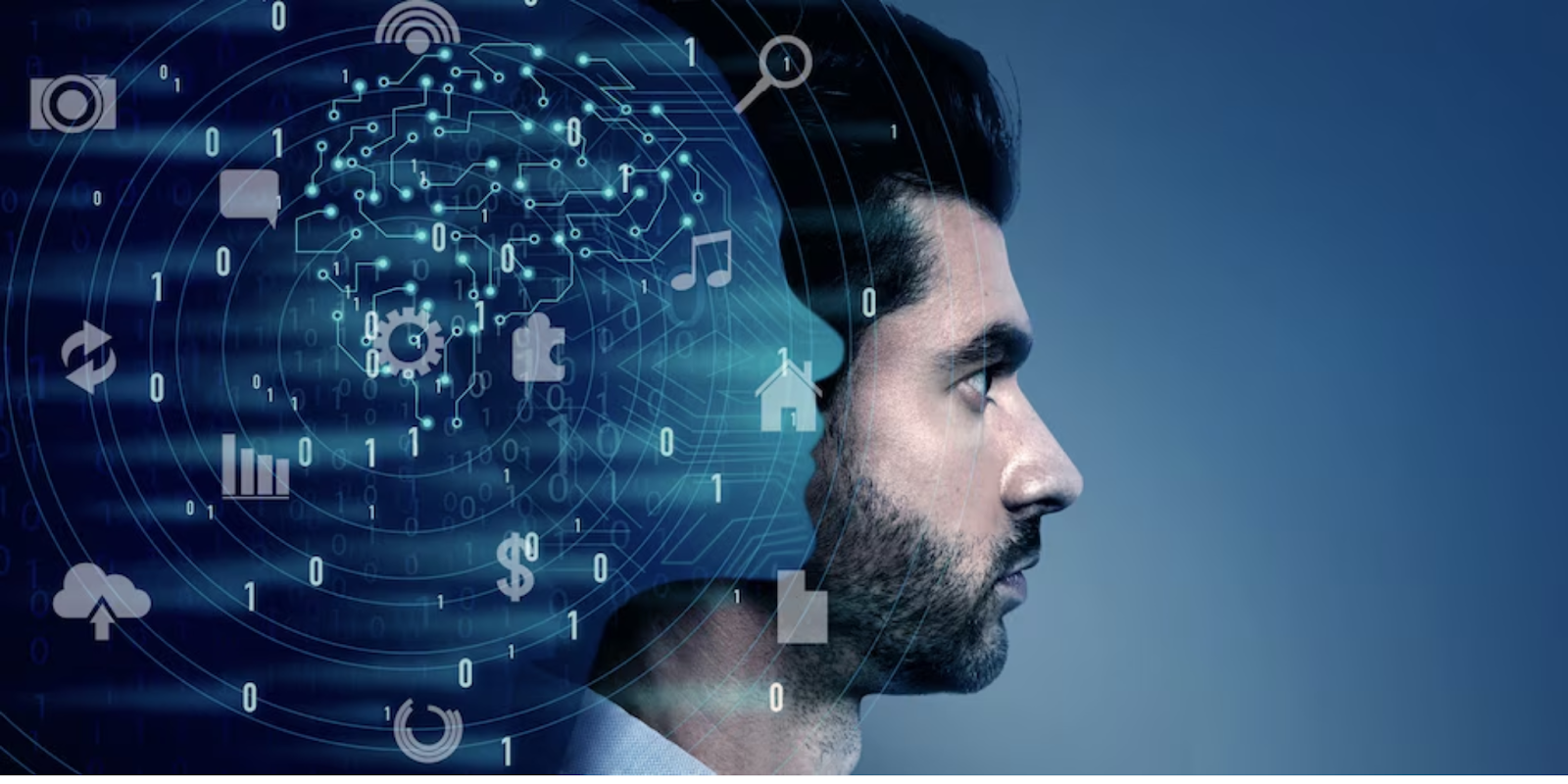
A generative AI model trained on thousands of images can create new, never-seen-before artwork by learning the patterns, styles, and elements from the data it has ingested. Similarly, it can be used in writing, music, video, or even more sophisticated processes like product design and automation.
How does generative AI work?
At its core, generative AI operates using machine learning models, specifically a class of algorithms known as Generative Adversarial Networks (GANs), Transformer models, and Variational Autoencoders (VAEs). These models are designed to generate new outputs by understanding and replicating the underlying structure of the input data.
Let’s break down the core processes:
1. Data collection and preprocessing
Before any AI can generate new content, it needs data to learn from. The first step in the generative AI process is data collection, where large amounts of data—whether text, images, or other types—are gathered. This data is then preprocessed to remove noise, normalize it, and prepare it for the model to learn from.
- Text data: For text generation, models may ingest books, articles, and other written content.
- Image data: For image generation, thousands of pictures are fed into the model for pattern recognition.
Why it matters: The quality of the generated output is directly linked to the quality of the input data. This is why data preprocessing is a critical step. Clean, relevant data leads to more accurate and innovative outputs.
2. Training the AI model
Once the data is preprocessed, it’s time to train the AI model. The training process typically involves machine learning algorithms like GANs or Transformer models (like GPT for text generation). These models learn from the input data by identifying patterns, understanding relationships between different data points, and building a representation of how elements interact.
- Generative adversarial networks (GANs): In a GAN model, there are two networks—the generator and the discriminator. The generator creates new data, while the discriminator evaluates whether the generated data is real or fake. The two networks work together to improve the quality of the generated data.
- Transformer models: Transformers, like GPT (Generative Pretrained Transformer), work especially well for natural language processing tasks. They leverage large amounts of text data to predict and generate new text, imitating human-like language.
3. Generation phase: creating new outputs
Once the model is trained, it can enter the generation phase, where it begins to create new content based on the patterns it has learned. The model is prompted with an initial input, and from there, it generates entirely new outputs—whether it’s generating text in response to a user query or creating new images based on a style it has learned.
Examples:
- Text: A generative AI model can complete paragraphs of text or even write entire articles based on prompts.
- Images: Models like DALL-E can generate detailed images from text descriptions.
- Audio/video: AI models can even generate lifelike audio, music, or video content.
4. Fine-tuning and iteration
The outputs generated by the AI models may not always be perfect on the first try. Therefore, a critical part of how generative AI works is fine-tuning the model based on feedback or additional training. The model learns from its mistakes and iterates to improve the quality of its outputs.
For example, if a model generates a piece of content that doesn’t meet the required quality, developers can adjust the model’s parameters or retrain it on more refined data.
5. Continuous learning and improvement
Generative AI models are not static. They continuously learn from new data and user feedback, improving their accuracy and quality over time. This continuous learning is critical for generative AI to stay relevant, especially in business applications where new data is constantly being generated.
Generative AI also benefits from transfer learning, where a model trained on one task can adapt and be used for another similar task, speeding up the deployment of new applications.
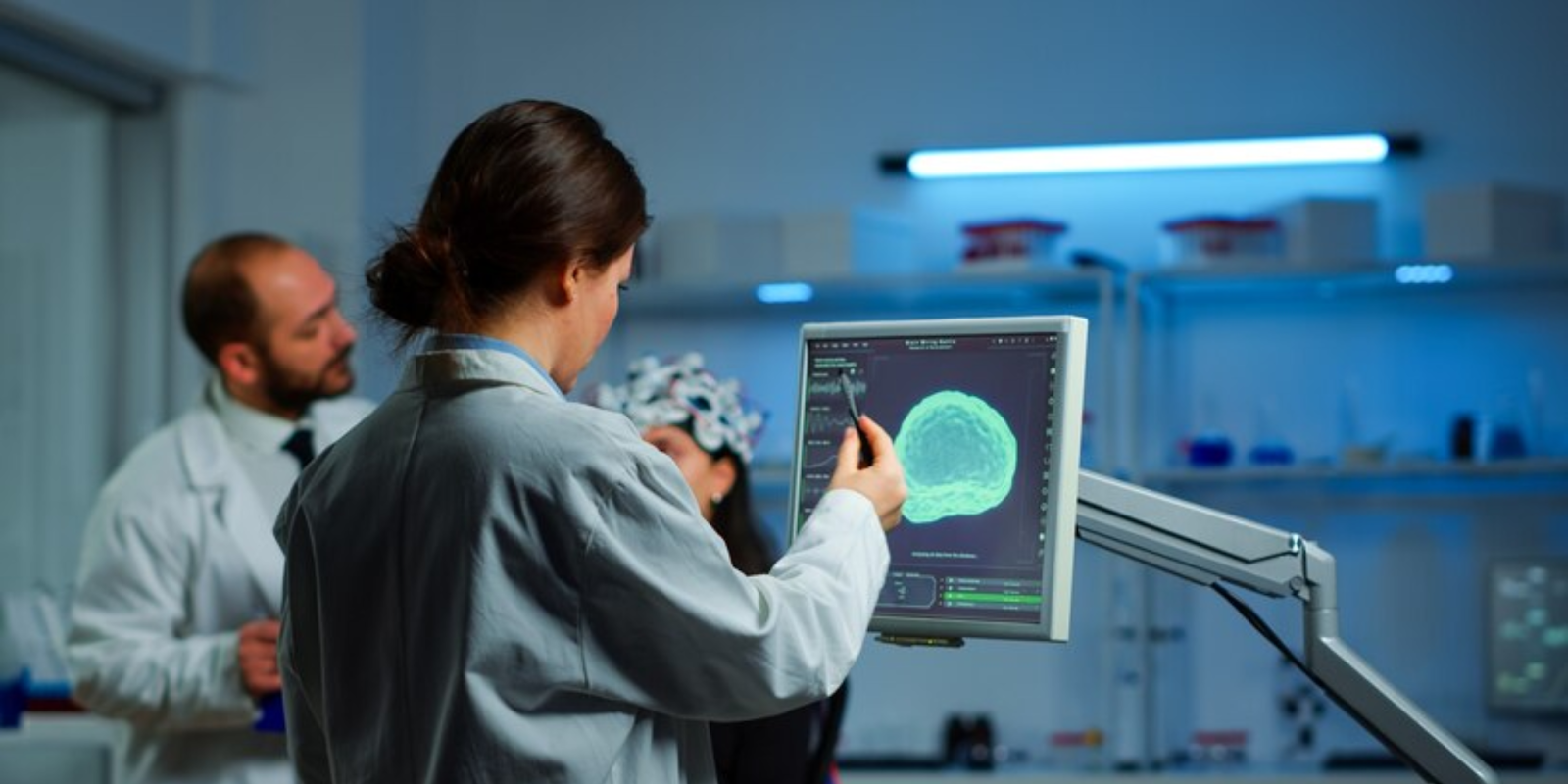
How generative AI works in practice
Generative AI is driving innovation across industries. Here's how it functions in some key areas:
1. Content creation in marketing
Generative AI is used to create personalized marketing content at scale. Whether it’s generating tailored email campaigns, producing social media posts, or creating engaging advertisements, AI can streamline creative processes.
A large e-commerce company uses generative AI to generate personalized product recommendations and marketing emails for millions of users, boosting engagement rates by 30%.
2. Product design and prototyping
In product development, generative AI helps designers create product prototypes faster by simulating different designs based on user preferences or market trends.
A major auto manufacturer used generative AI to design vehicle components, reducing the time needed to create and test new parts by 50%.
3. Healthcare: drug discovery
In healthcare, generative AI helps with drug discovery by simulating molecular structures and predicting their effectiveness, drastically reducing the time it takes to discover new drugs.
A pharmaceutical company used generative AI to explore potential COVID-19 treatments, reducing research time by months.
Challenges and considerations
Despite its potential, generative AI comes with its own set of challenges. For businesses looking to adopt generative AI, it’s crucial to understand these limitations:
1. Data quality
Generative AI models are only as good as the data they are trained on. Poor-quality data can lead to inaccurate or biased outputs, which can be detrimental in applications like healthcare or finance.
2. Ethical considerations
Generative AI has the potential to create deep fakes, biased content, or misleading information. Businesses need to incorporate ethical guidelines when using AI to ensure responsible deployment.
Learn more about the challenges generative AI faces in our blog on what challenges does generative AI face with respect to data.
3. Infrastructure requirements
For generative AI to work at scale, businesses need robust cloud and data infrastructure capable of handling large datasets and computational demands. This requires significant investment in cloud modernization and database optimization.
Explore how our cloud modernization services can help prepare your enterprise for AI scalability.
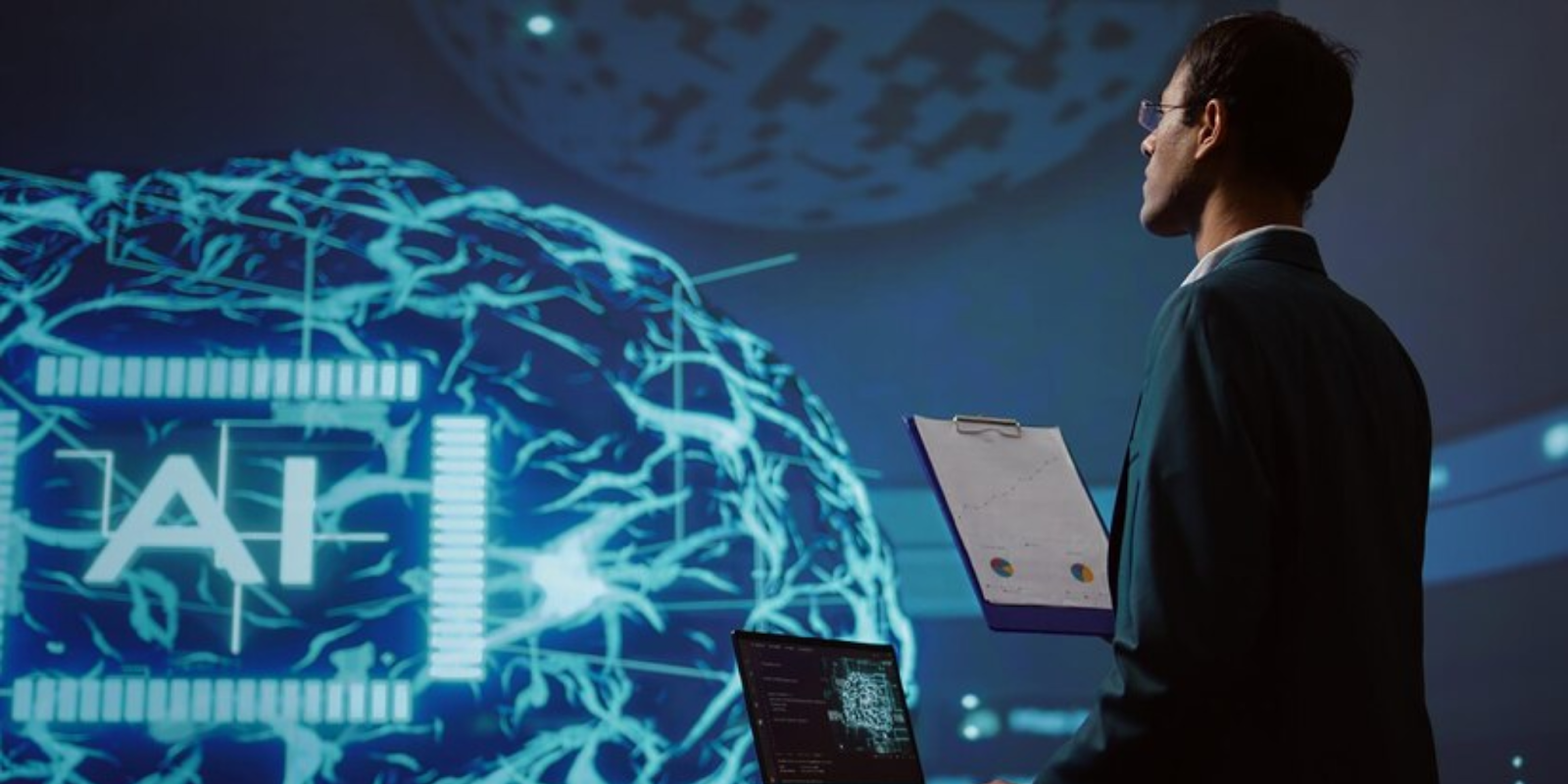
Generative AI—a transformative force in innovation
Generative AI is revolutionizing industries by enabling businesses to create new content, innovate faster, and solve complex problems. From marketing to product design to healthcare, the ability to generate new outputs based on existing data is transforming how businesses operate.
While the technology is powerful, it’s essential for enterprises to have the right data, infrastructure, and ethical considerations in place to fully harness its potential.
Ready to explore how generative AI can transform your business? Get in touch with us for a free expert consultation to learn more about our custom generative AI solutions and see how we can help you drive innovation and ROI.