Generative AI is revolutionizing industries, offering transformative capabilities from automating content creation to enhancing decision-making processes. However, as enterprises increasingly adopt Generative AI, they encounter significant challenges, particularly concerning data.
Read to know more about the role of data in generative AI and their interplay.
This blog explores the journey from understanding these challenges to implementing forward-looking solutions that ensure successful and secure Generative AI deployments. We will read into data privacy, security, and the broader adoption landscape, providing a comprehensive guide to understand this evolving field.
Laying the Groundwork

The adoption of Generative AI is not a linear process. Enterprises must navigate a complex curve that includes initial experimentation, scaling successful initiatives, and achieving widespread deployment. Understanding where your organization stands on this adoption curve is crucial to overcoming the data-related challenges that lie ahead.
Key Points
- Generative AI Adoption Rate: The pace at which Generative AI is being integrated into enterprises is accelerating, but it varies significantly across industries.
- Strategic Alignment: Enterprises must align their AI initiatives with business goals to ensure that adoption drives tangible outcomes.
73% of NextGen believe that generative AI is a powerful force for transformation.
By understanding the Generative AI adoption curve, organizations can better anticipate the challenges ahead and position themselves for successful scaling.
Safeguarding the Foundation
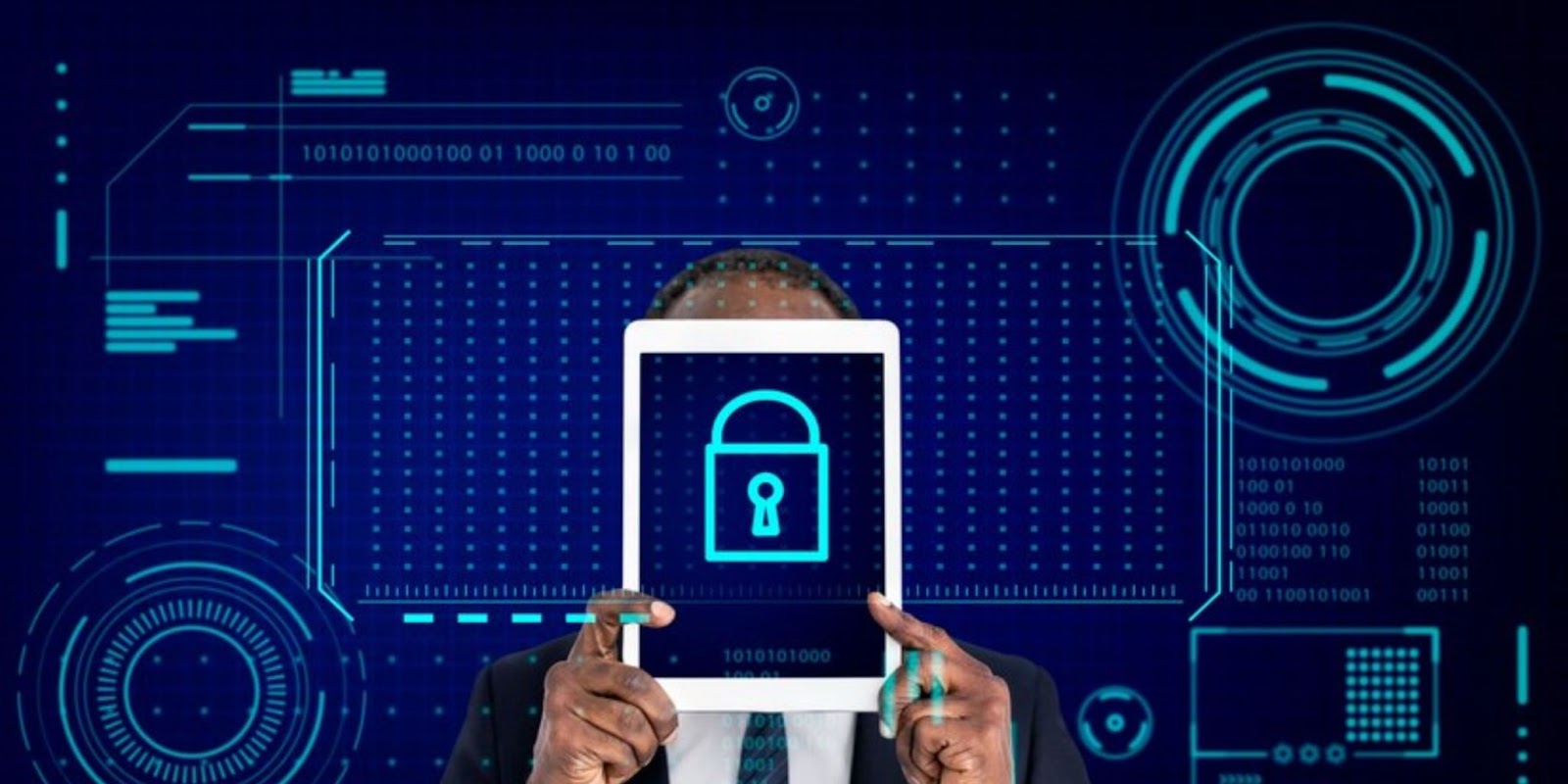
As enterprises embrace Generative AI, safeguarding data privacy and security becomes paramount. Generative AI systems rely on vast amounts of data, raising concerns about data protection and compliance with regulations such as GDPR and CCPA.
Key Challenges
- Data Privacy in Generative AI: Ensuring that AI systems respect user privacy and handle personal data responsibly is critical.
- Generative AI Data Security: Protecting AI models from data breaches and cyber threats is essential to maintaining trust and operational integrity.
What Can Be Done
- Robust Encryption and Anonymization: Implement strong encryption protocols and data anonymization techniques to protect sensitive information.
- Compliance Monitoring: Regularly audit AI systems to ensure compliance with relevant data protection regulations.
Addressing data privacy and security is not just a regulatory requirement but a strategic imperative that builds trust and ensures the long-term viability of Generative AI solutions.
Building Reliable AI
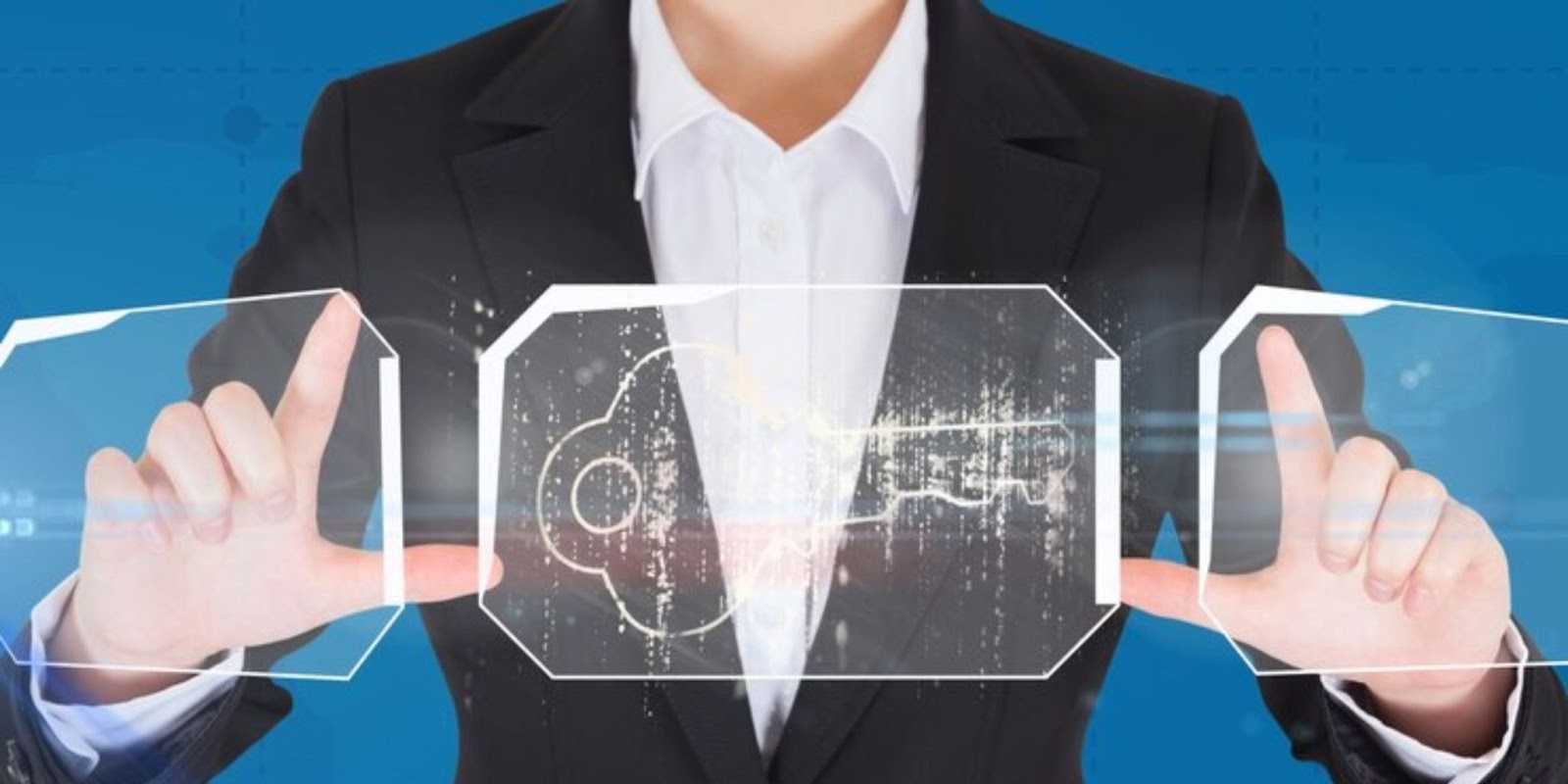
The success of Generative AI hinges on the quality and integrity of the data it processes. Poor data quality can lead to unreliable AI outputs, undermining the value that these systems can deliver to enterprises.
Key Challenges
- Data Quality: Ensuring that data is accurate, complete, and relevant is crucial for training effective AI models.
- Data Integrity: Maintaining the consistency and reliability of data across the AI lifecycle is essential for producing trustworthy results.
What Can Be Done
- Data Governance Frameworks: Implement comprehensive data governance practices that standardize data quality management across the organization.
- Continuous Data Validation: Regularly validate and cleanse data to prevent errors from propagating through AI models.
Investing in data quality and integrity is essential for building reliable and effective Generative AI systems that deliver consistent value.
Ethical Complexities of Responsible AI

Generative AI presents unique ethical challenges, particularly around bias and fairness. Ensuring that AI systems operate in an ethical manner is crucial for maintaining public trust and achieving equitable outcomes.
Key Challenges
- Bias in AI Models: Data used to train AI models can introduce biases that lead to unfair or discriminatory outcomes.
- Synthetic Data and Ethics: While synthetic data can enhance AI capabilities, it also raises ethical questions about accuracy and representation.
What Can Be Done
- Ethical AI Frameworks: Develop and implement ethical guidelines that address bias, fairness, and transparency in AI systems.
- Diverse Data Sources: Use diverse datasets to train AI models, reducing the risk of biased outcomes.
Addressing ethical challenges in Generative AI is essential for building systems that are not only innovative but also socially responsible.
Scaling Generative AI Across the Enterprise
To fully realize the potential of Generative AI, enterprises must move beyond pilot projects and scale their AI initiatives across the organization. This requires a forward-looking approach that integrates AI with existing business processes and drives continuous innovation.
Key Strategies
- Enterprise-Wide Integration: Seamlessly integrate AI into core business processes to unlock new efficiencies and insights.
- Continuous Improvement: Foster a culture of innovation that encourages the ongoing refinement of AI models and strategies.
By adopting a strategic approach to scaling Generative AI, enterprises can drive sustained growth and innovation, positioning themselves as leaders in the digital economy.
Building a Future-Ready Generative AI Strategy
As Generative AI continues to evolve, enterprises must navigate a complex landscape of data-related challenges to achieve successful adoption. By addressing data privacy, security, quality, and ethical considerations, organizations can build robust AI systems that deliver meaningful value. A forward-looking approach that aligns AI initiatives with business objectives and fosters continuous innovation is key to unlocking the full potential of Generative AI.
Discover how we as a generative AI development services company can help you navigate these challenges and scale Generative AI across your enterprise: Generative AI Development Services Company.