According to a report by MarketsandMarkets, the global advanced analytics market is projected to reach $29.53 billion by 2024, growing at a CAGR of 21.6%. This blog helps in understanding the complex concepts related to advanced analytics, its types, applications, and benefits, helping you understand how it can revolutionize your business operations.
Think you know the core concepts of Advanced Analytics? Let’s jump to the section discussing its role in Predictive Modeling!
Introduction to Advanced Analytics
Advanced analytics encompasses a range of sophisticated techniques and tools designed to analyze complex data sets. Unlike traditional analytics, which focuses on historical data, advanced analytics leverages statistical methods, machine learning, and predictive modeling to uncover patterns, forecast future trends, and provide actionable insights.
Advanced analytics goes beyond traditional data analysis by applying complex algorithms and sophisticated techniques to uncover deeper insights and predict future outcomes. It involves the use of data mining, machine learning, predictive modeling, and artificial intelligence to analyze data and provide actionable insights.
This advanced approach enables businesses to not only understand what has happened in the past but also to anticipate future trends and make data-driven decisions.
Understanding these three types of analytics helps businesses leverage their data more effectively, leading to better decision-making and strategic planning.
Types of Advanced Analytics:
There are several types of advanced analytics, each serving a specific purpose and providing unique insights.
- Descriptive Analytics
Descriptive analytics focuses on summarizing historical data to understand what has happened. It involves the use of data aggregation and data mining techniques to provide insights into past events and identify patterns and trends. This type of analytics is essential for understanding the context and background of data, which helps in making informed decisions.
Example
A retail company uses descriptive analytics to analyze sales data from the past year. By summarizing the data, they can identify which products were the best sellers, which months had the highest sales, and what customer demographics contributed to the sales.
- Predictive Analytics
Predictive analytics uses historical data to predict future outcomes. By applying statistical models, machine learning algorithms, and data mining techniques, predictive analytics can forecast trends, identify potential risks, and anticipate customer behavior. This type of analytics is crucial for planning and decision-making, as it allows businesses to prepare for future scenarios.
Example
A financial institution uses predictive analytics to assess the credit risk of loan applicants. By analyzing historical data on past loan repayments and default rates, the institution can predict the likelihood of a new applicant defaulting on a loan.
- Prescriptive Analytics
Prescriptive analytics goes beyond prediction by recommending specific actions to achieve desired outcomes. It uses optimization algorithms, simulation techniques, and machine learning to provide actionable insights and suggest the best course of action. This type of analytics helps businesses make data-driven decisions that align with their strategic goals.
Example
A logistics company uses prescriptive analytics to optimize delivery routes. By analyzing data on traffic patterns, delivery times, and fuel consumption, the company can identify the most efficient routes and reduce operational costs.
- Diagnostic Analytics
Diagnostic analytics focuses on identifying the root causes of past events. It involves drilling down into data to understand why something happened. This type of analytics is essential for problem-solving and improving processes.
Example
A manufacturing company uses diagnostic analytics to investigate a sudden drop in production. By analyzing data from different stages of the production process, they can identify the cause of the issue and take corrective actions.
- Cognitive Analytics
Cognitive analytics mimics human thought processes to analyze data. It uses artificial intelligence, natural language processing, and machine learning to understand and interpret complex data. This type of analytics is useful for handling unstructured data and making sense of large volumes of information.
Example
A healthcare provider uses cognitive analytics to analyze patient records and research papers. By understanding the context and extracting relevant information, the provider can improve patient care and treatment outcomes.
Understanding the different types of advanced analytics helps businesses choose the right approach for their specific needs. Each type offers unique insights and capabilities, enabling organizations to harness the full potential of their data.
Applications of Advanced Analytics

Advanced analytics is applied across various industries to solve complex problems and improve operational efficiency.
- Healthcare
In healthcare, advanced analytics is used to predict patient outcomes, optimize treatment plans, and improve operational efficiency. By analyzing patient data, healthcare providers can identify high-risk patients, predict disease outbreaks, and personalize treatment plans.
- Finance
In the financial industry, advanced analytics is used for fraud detection, risk management, and investment strategies. By analyzing transaction data, financial institutions can identify suspicious activities, assess credit risk, and optimize investment portfolios.
- Retail
In retail, advanced analytics is used for customer segmentation, demand forecasting, and inventory management. By analyzing customer data, retailers can personalize marketing campaigns, optimize inventory levels, and improve the overall customer experience.
- Manufacturing
In manufacturing, advanced analytics is used for predictive maintenance, quality control, and supply chain optimization. By analyzing sensor data and production metrics, manufacturers can predict equipment failures, improve product quality, and streamline supply chain operations.
- Marketing
In marketing, advanced analytics is used for customer insights, campaign optimization, and performance measurement. By analyzing marketing data, businesses can understand customer preferences, optimize marketing strategies, and measure the effectiveness of campaigns.
The role of advanced analytics experts are vast and varied, offering significant benefits to different sectors. By leveraging advanced analytics, businesses can solve complex problems, improve operational efficiency, and drive strategic growth.
Benefits of Advanced Analytics
The adoption of advanced analytics provides numerous advantages that can transform business operations.
- Improved Decision Making
Advanced analytics provides data-driven insights that enable businesses to make informed decisions. By understanding past trends, predicting future outcomes, and recommending actions, advanced analytics helps businesses navigate uncertainties and make strategic decisions.
- Operational Efficiency
Advanced analytics optimizes business processes, reduces costs, and improves efficiency. By analyzing data from various sources, businesses can identify inefficiencies, streamline operations, and enhance productivity.
- Enhanced Customer Experience:
Advanced analytics helps businesses personalize interactions and improve customer satisfaction. By analyzing customer data, businesses can understand customer preferences, anticipate needs, and deliver personalized experiences.
- Competitive Advantage
Advanced analytics provides a competitive edge by identifying market trends, opportunities, and threats. By leveraging advanced analytics, businesses can stay ahead of competitors, adapt to changing market conditions, and seize new opportunities.
- Risk Management
Advanced analytics helps businesses identify and mitigate risks. By analyzing data from various sources, businesses can detect anomalies, assess risks, and implement preventive measures.
Leveraging advanced analytics enables businesses to enhance performance, drive growth, and stay ahead of the competition. The benefits of advanced analytics extend across various aspects of business operations, providing valuable insights and improving decision-making processes.
Essential Infrastructure Technologies Required for Advanced Analytics
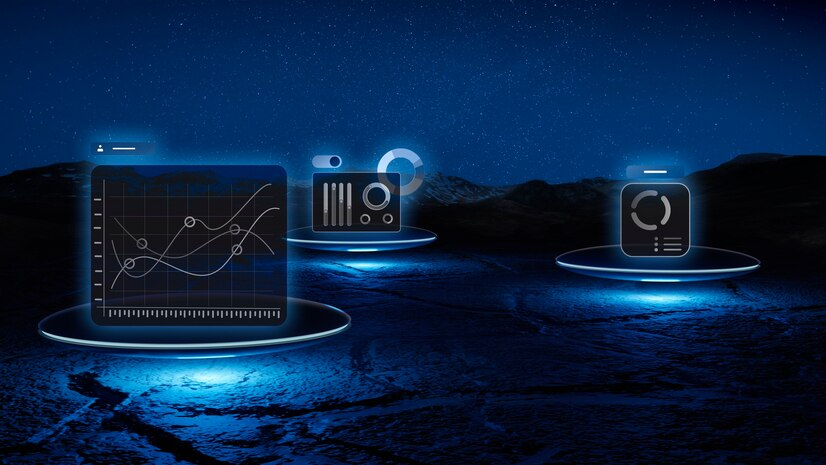
Implementing advanced analytics requires a robust infrastructure capable of handling vast amounts of data and complex computations.
- Data Storage Solutions
Advanced analytics requires efficient and scalable data storage solutions to manage large volumes of data. Cloud-based storage options like Amazon S3 provide the necessary scalability and accessibility.
To know more about the Amazon S3 abilities, read how data lifecycle management can be done with Amazon S3 Intelligent-Tiering.
- Data Processing Frameworks
Data processing frameworks like AWS Lambda enable the processing of large data sets across distributed computing environments. These frameworks provide the necessary tools for batch and real-time data processing.
The use of AWS Lambda for serverless computing in SaaS is a must read for knowing its role!
- Machine Learning Platforms
Machine learning platforms like AWS Sagemaker provide the tools and libraries needed to develop, train, and deploy machine learning models.
How to Leverage Advanced Analytics for Predictive Modeling
Predictive modeling is a key application of advanced analytics that helps businesses forecast future trends and make proactive decisions. Leveraging advanced analytics for predictive modeling involves several steps and best practices.
- Data Collection and Preparation
The first step in predictive modeling is to collect and prepare the data. This involves gathering data from various sources, cleaning the data to remove inconsistencies, and transforming it into a suitable format for analysis.
- Feature Engineering
Feature engineering involves selecting and creating the variables (features) that will be used in the predictive model. This step is crucial for improving the accuracy and performance of the model.
- Model Selection and Training
The next step is to select the appropriate predictive modeling technique and train the model. Common techniques include regression analysis, decision trees, and neural networks.
- Model Evaluation
Once the model is trained, it is evaluated using various metrics such as accuracy, precision, recall, and F1 score. This step ensures that the model performs well on unseen data.
- Deployment and Monitoring
The final step is to deploy the model in a production environment and monitor its performance. This involves integrating the model into business processes and continuously monitoring its predictions to ensure accuracy.
Leveraging advanced analytics for predictive modeling involves a series of steps, from data collection and preparation to model deployment and monitoring. By following best practices and using the right techniques, businesses can create accurate predictive models that drive strategic decision-making.
Advanced Analytics and Cloud Management
Integrating advanced analytics with cloud management enhances scalability, accessibility, and efficiency.
- Scalability
Cloud platforms provide the necessary infrastructure to handle large volumes of data. By leveraging cloud services, businesses can scale their analytics capabilities based on demand and ensure efficient data processing.
- Real-Time Processing
Cloud-based analytics solutions enable real-time data analysis and insights. By processing data in real-time, businesses can make timely decisions, respond to changing conditions, and seize opportunities.
- Cost-Effectiveness
Cloud services offer flexible pricing models, reducing the costs associated with data storage and processing. By paying for only the resources used, businesses can optimize their budgets and reduce operational costs.
Integrating advanced analytics with cloud management provides businesses with the scalability, real-time processing capabilities, and cost-effectiveness needed to handle vast amounts of data efficiently. This synergy enhances the ability to make timely, informed decisions, ultimately driving business growth and innovation.
Future Trends in Advanced Analytics
As technology continues to evolve, advanced analytics is poised to play an increasingly significant role in business operations. Emerging trends in this field are set to redefine how organizations leverage data for strategic advantage.
- Artificial Intelligence and Machine Learning
The integration of AI and machine learning with advanced analytics is expected to enhance predictive accuracy and provide deeper insights. These technologies enable the automation of complex data analysis processes, making it easier to uncover hidden patterns and predict future trends.
- Real-Time Analytics
The demand for real-time data processing and analytics is growing as businesses seek to make immediate, data-driven decisions. Real-time analytics allows organizations to respond swiftly to market changes, customer behaviors, and operational challenges.
- Edge Computing
Edge computing brings data processing closer to the source of data generation, reducing latency and improving the speed of analytics. This trend is particularly relevant for industries that rely on IoT devices and require rapid data analysis.
The future of advanced analytics is marked by the integration of AI and machine learning, the rise of real-time analytics, and the adoption of edge computing. These trends will further empower businesses to harness the full potential of their data, driving innovation and competitive advantage.
The Takeaway
Advanced analytics is a powerful tool that can transform how businesses operate and make decisions. By leveraging sophisticated techniques and cloud management solutions, organizations can gain deeper insights, predict future trends, and optimize their operations. As the market continues to grow, the importance of advanced analytics will only increase, making it a critical component of any successful business strategy.
Ready to harness the power of advanced analytics? It’s time to book a free cloud-expert consultation and discover how our advanced analytics solutions can drive your business forward.