Generative AI’s capabilities are deeply intertwined with the quality of data it processes. The effectiveness of AI models like GPT hinges on diverse, high-quality datasets that allow the AI to learn and adapt to complex patterns. Companies that focus on curating extensive datasets can significantly enhance the relevance and accuracy of AI outputs.

This blog delves into the critical aspects of how data impacts generative AI, the types of data best suited for these models, and the strategies needed to ensure data quality and governance in generative AI systems.
Data as the Foundation for Generative AI

What is the Role of Data in Generative AI?
Generative AI relies heavily on data to function. These models, such as GPT (Generative Pre-trained Transformer), need vast amounts of data to learn patterns, structures, and relationships within the information they process. The quality, volume, and diversity of this data directly influence the performance of generative AI systems.
For instance, models trained on diverse datasets can produce more accurate and contextually relevant outputs, enhancing their applicability across various domains.
According to Capgemini, the effectiveness of generative AI is only as strong as the data it is trained on. Companies that invest in high-quality, diverse datasets have seen significantly better AI performance compared to those relying on narrow or biased data sources.
Where Does Generative AI Get Its Data?
Generative AI models acquire data from a variety of sources. This includes structured data like databases and spreadsheets, as well as unstructured data such as text, images, and videos. The choice of data sources depends largely on the intended application of the AI model.
For example, a model designed to generate human-like text would need extensive text datasets from books, articles, and online content.
The type and quality of data fed into generative AI models are crucial determinants of their output's relevance and accuracy. Businesses must carefully curate their data sources to maximize the potential of generative AI.
Optimal Data Types for AI Generation

What Type of Data is Generative AI Most Suitable For?
Generative AI is versatile and can work with both structured and unstructured data. However, it is particularly powerful when dealing with unstructured data, such as natural language, images, and audio. This type of data is rich in information and allows AI models to create complex and nuanced outputs.
Research from AWS highlights that companies utilizing a blend of structured and unstructured data in their AI models have unlocked deeper insights and achieved more nuanced decision-making processes.
The development of an AI-powered remote patient monitoring system is a recent practical implementation. This system was designed to continuously track and analyze patient data from various sources, including wearable devices and medical records. By processing this unstructured data in real-time, the system provided healthcare professionals with actionable insights, significantly improving patient outcomes.
This example underscores how generative AI can be harnessed to handle complex, unstructured data for impactful results, particularly in fields requiring real-time decision-making and analysis.
Generative AI for Structured vs. Unstructured Data
While generative AI can process structured data effectively, its real strength lies in its ability to handle unstructured data. For instance, AI models can generate realistic images, translate languages, and even create music by learning from vast collections of unstructured datasets.
On the other hand, structured data is typically used for more straightforward tasks, like filling in missing values or generating predictive analytics.
Generative AI's capability to process and generate outputs from unstructured data sets it apart from traditional AI models, making it a valuable tool in fields requiring creative and complex outputs.
Maintaining Data Integrity for Generative AI

Data Quality in Generative AI
The quality of data is a critical factor in the success of generative AI models. Poor-quality data can lead to biased, inaccurate, or irrelevant outputs, which can have significant implications, especially in industries like healthcare and finance. Ensuring data quality involves rigorous cleaning, validation, and processing to eliminate errors and inconsistencies.
A study published in Harvard Business Review indicates that companies with robust data governance frameworks see up to 30% improvement in AI model accuracy due to enhanced data quality.
Data Governance for Generative AI
Data governance refers to the management framework that ensures data's availability, usability, integrity, and security within an organization. For generative AI, data governance is crucial not only to maintain high data quality but also to comply with regulatory standards, protect privacy, and prevent biases in AI models.
Implementing strong data governance practices is essential for maintaining the integrity and reliability of generative AI outputs, ensuring that the AI operates ethically and within regulatory frameworks.
Enhancing AI Models with Your Company’s Data
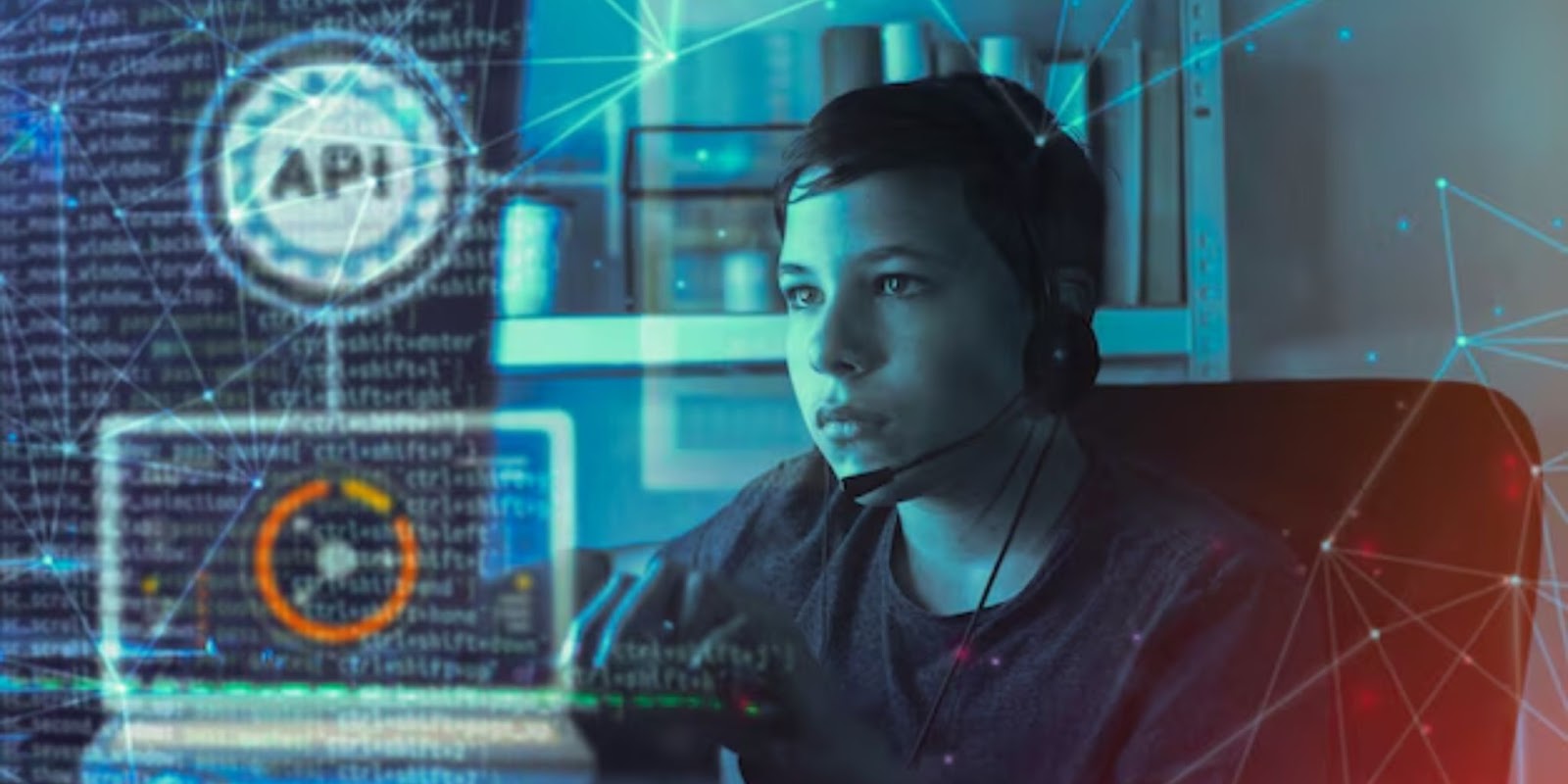
How to Train Generative AI Using Your Company’s Data
Training generative AI using your company's data involves several critical steps. First, you need to gather and prepare the data, ensuring it is clean, relevant, and representative of the tasks you want the AI to perform. Next, you need to select the appropriate model architecture and configure it to learn from the data effectively.
This process often requires a balance between feeding the AI enough data to learn without overwhelming it with irrelevant information.
AWS has noted that businesses that align their data strategy with their AI objectives tend to see a 25% increase in operational efficiency, demonstrating the importance of strategic data preparation.
Training generative AI models with your own data can give your business a competitive edge, as the AI can generate outputs that are highly tailored to your specific needs. However, the process requires careful planning, robust data governance, and a clear understanding of the types of data that will be most beneficial for your objectives.
A New Era in Data Analytics
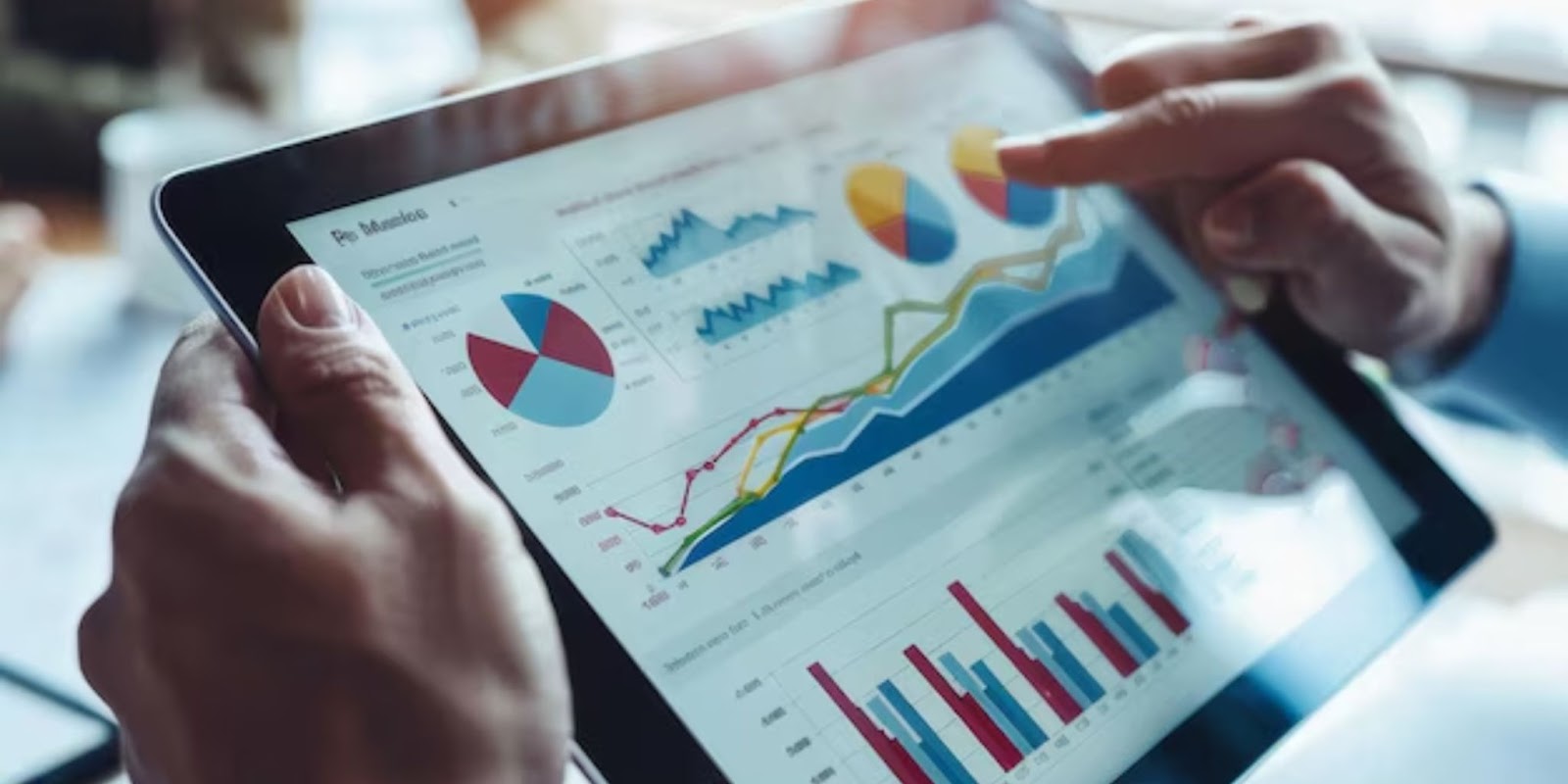
Generative AI and Data Analytics
Generative AI enhances data analytics by not just analyzing existing data, but also by generating new data patterns and insights. This capability is particularly useful in predictive analytics, where AI models can forecast trends and behaviors based on learned data patterns.
According to recent data from Capgemini, companies that have integrated generative AI into their data analytics processes have experienced a 40% improvement in their ability to predict market trends and consumer behavior.
Generative AI in Data Management
Generative AI can also streamline data management by automating tasks such as data cleaning, integration, and transformation. This not only reduces the time and effort required for data management but also minimizes the risk of human error, leading to more accurate data handling.
The integration of generative AI into data analytics and management processes offers significant advantages, from improved predictive capabilities to more efficient data handling. For businesses looking to stay ahead in a data-driven world, generative AI is an essential tool.
Final Thoughts
The role of data in generative AI development is multifaceted and crucial. From determining the quality of AI outputs to guiding the training process and enhancing data analytics, data is the cornerstone of generative AI. As businesses increasingly adopt generative AI, understanding how to manage, prepare, and govern data effectively will be key to unlocking the full potential of this transformative technology.
As generative AI continues to evolve, so too must our approaches to data management and governance. By investing in high-quality data and robust governance frameworks, businesses can ensure that their AI models deliver reliable, accurate, and valuable results, driving success in an increasingly competitive market.
Unlock the Power of Data with Generative AI
If you're looking to take your business to the next level with generative AI, partnering with experts who understand the importance of data in AI development is essential. Explore how a generative AI development services company can help you leverage cutting-edge AI solutions tailored to your needs.
Get in touch with us today and transform your data into actionable insights and innovative solutions with our specialized services.